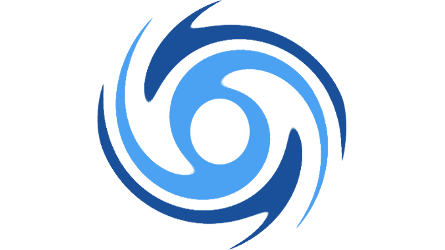
Steem Sincerity is a project aimed at helping to address the spam problem we have on Steem.
As I explained in my introductory post there are three aspects to this. This post discusses the most important aspect in more detail.
Public API for Developers
This is a service hosted on my server(s), which can be queried by any front-end website or app to obtain information about Steem accounts. It uses a database which stores the last 7 days worth of posts, comments and votes.
Periodically the software extracts meta-data (data about the data) from these accounts, and much of this can be easily accessed by application developers using the methods here. The meta-data for each account is also fed into a kind of artificial intelligence software which looks at how it compares to other known spamming and bot accounts, so it can 'classify' each active account.
What is classification?
In machine learning, classification is an approach in which the computer program learns from the data input given to it and then uses this learning to classify new observations. So from our perspective, we first 'train' the classifier by giving it three lists of Steem accounts that have been manually classified as either Human Content Creator, Spammer or Bot.
It is programmed to be able to extract the relevent meta-data - or what are called features in machine learning - from these accounts. Some of the many features used in the Steem Sincerity software are: number of comments, number of posts, average number of downvoted comments, average word length etc. It looks at how these features vary between the different classes of account, and makes rules for itself to use when deciding about how to classify accounts that it hasn't seen before.
The classifier has currently been trained using only around 30 accounts of each type, and has a cross-validation accuracy of around 78%, and very little non-spam is classified as spam. Cross-validation is a standard technique for evaluating the accuracy of a classifier, but of course what constitutes spam is highly personal, so inevitably my preferences will have introduced biases. A larger crowdsourced training set is planned to reduce this bias in the near future.
Rather than making a direct prediction about whether an account belongs to a spammer, the API actually returns the probabilities of the account belonging to each of the three classes. For example an account may show the following classifications scores:
Human Content Creator: 45%
Spammer: 45%
Bot: 10%
Each front-end using the API can make its own decision about what should happen at different spam thresholds. For example, it could fade the comment if the spammer score is between 40-70% and hide it altogether if the score exceeds 70%. It could even leave this up to the user to decide.
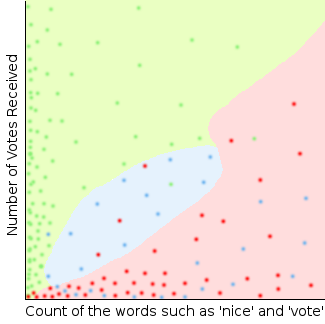
This is a very simple illustration of how accounts with comments containing certain combinations of features may be classified as spam. The red dots represent real spamming accounts, and the pink area shows the accounts which are classified as spammers. The accuracy is not perfect, but good enough to be useful. In practice the machine learning algorithm used by the Sincerity software uses far too many features to be able to show in a two-dimensional diagram.
API Specifications
If you are a developer, you can find the API specification here. There are currently 10 methods, and since the main intention is to help improve front-end user experiences, performance is prioritised over the having larger amounts of historical data. Currently no API keys are required, and request rate limiting is fairly relaxed, but this may need to change depending on future demand.
Main Methods
/api/accounts-info/account1,account2,account2
This expects a comma separated list of accounts, and returns various useful meta-data about the accounts. This includes the probability that each account is a: Human Content Creator, Spammer or Bot. It also includes some metrics about the commenting and voting behaviour of the accounts. Note that only accounts which have commented in the last period will have records in the database. Because up to 100 accounts can be queried at a time, this is the most useful method for hiding or changing the appearance of spam in your application.
/account-full-info/account1
This returns the complete analysis information that are held for the account specified. There are many fields, a few of which are unused. You may want to query this when an account profile is clicked for example.
/account-comments/account1
Returns a time-sorted list of the comments made by the specified account in the last 7 days.
/account-outgoing-votes/account1
Returns a time-sorted list of the votes made by the specified account in the last 7 days.
/account-outgoing-downvotes/account1
Returns a time-sorted list of the flags given by the specified account in the last 7 days.
/account-apps-used/account1
Returns the list of apps the specified user has used to post and comment in the last 7 days.
/biggest-spammers/
Returns the 500 accounts most likely to be spamming accounts. This may be useful for stakeholders employing bots to clean up the platform.
There are a few other methods, and I will add more over time.
I'll be improving the Chrome Sincerity extension soon, to use some of these new methods.
If you have other requirements for a different API method or need to apply machine learning to different data, I'd be delighted to work for STEEM ;)